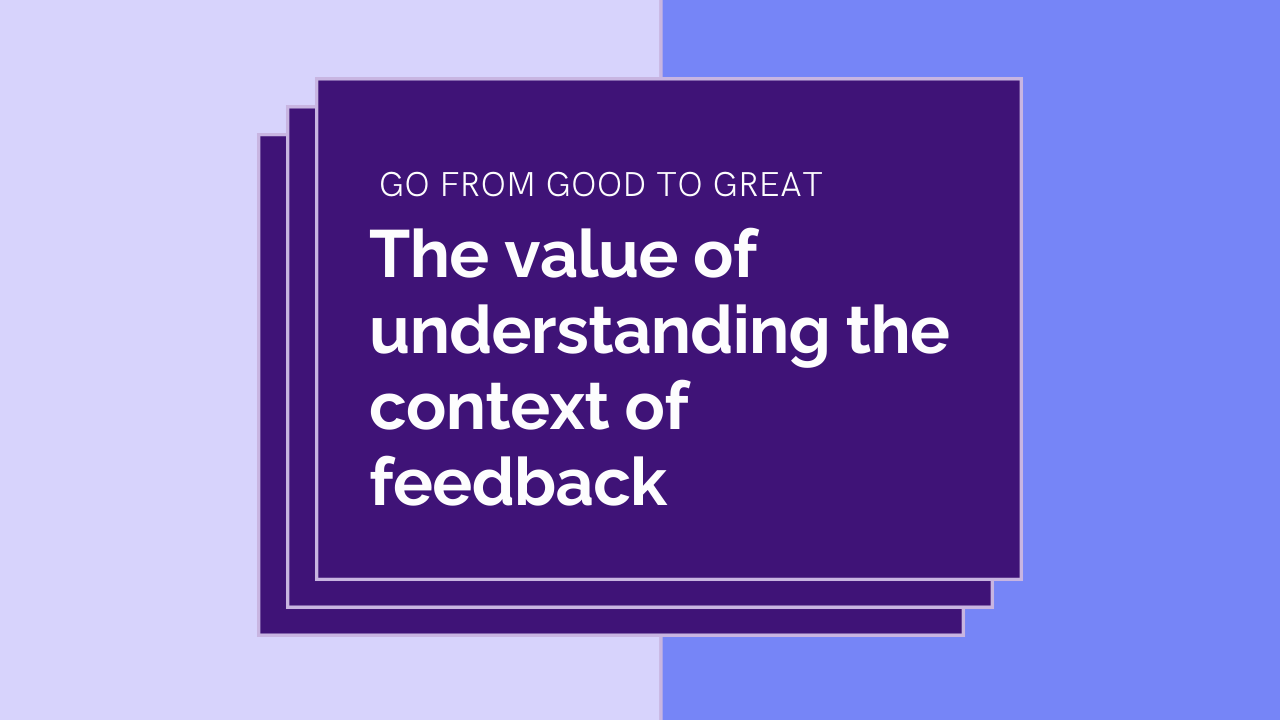
Go from Good to Great: The Value of Understanding Feedback Context
.webp)
AI has changed the game of big data analysis in many spaces. Understanding the Voice of Customer is one space where the change is particularly monumental. Brands no longer need to rely on a manually created list of tags applied haphazardly (at worst) or unevenly (at best) to feedback records by resources with natural and unique biases.
Today, both off-the-shelf and custom Large Language Models can serve as the bulwark for tagging and categorizing feedback records, alleviating both the need for manual tagging and, more importantly, the inherent degradation of analysis quality.
Unfortunately, while automating the process of manually tagging feedback has alleviated many problems, it has also led to blind spots in understanding and, ultimately a deep misunderstanding of what your customers are telling you in these valuable records.
Limits of analyzing feedback by keywords
In real-world conversation, our brains are programmed to recognize nuance. Unfortunately, the majority of feedback analytics tools are calibrated to organize records by keyword, often called a “topic.”
For example, a client may share product feedback that looks like this:
"Love the overall UI of the app, but been struggling with the slow download speeds of albums. I also wish they would release a dark mode in the future for their Android app just like the web version"
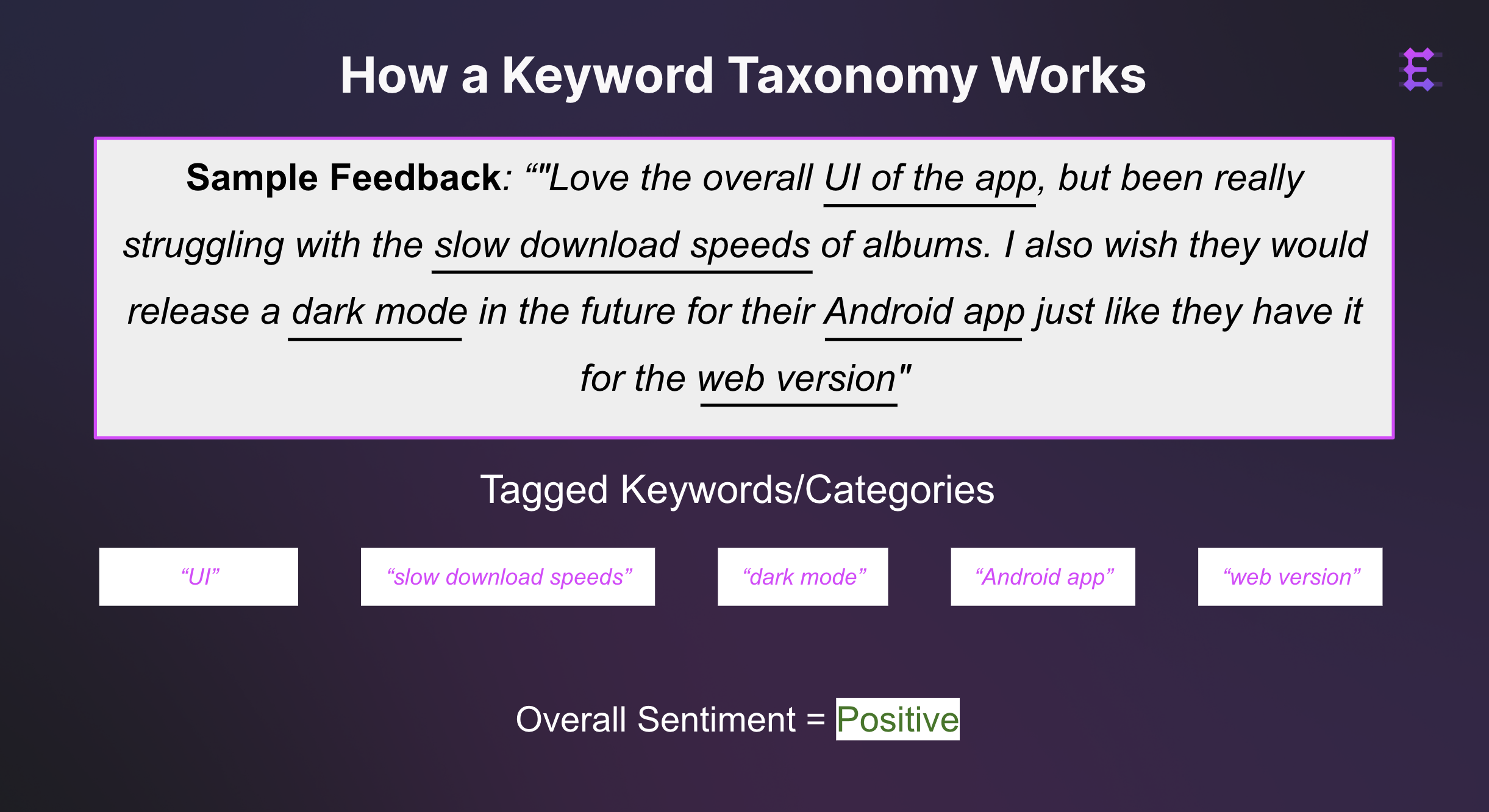
In this case, a model may extract keywords like:
- “UI of the app”
- “slow download speeds”
- “dark mode”
- “Android app”
- “web version”
Furthermore, because the record begins with praise, the sentiment level may be tagged as Positive, effectively overlooking the rest of the context of the record.
If you are digging into the data, would any of those tagged Topics lead you to a conclusion short of further mining and manually digging? How would the topics “dark mode” or “web version” point you to a useful next step? For anyone reviewing this data as part of a larger data set, they would be highly likely to reach unsubstantiated conclusions.
Deeply understand feedback using context
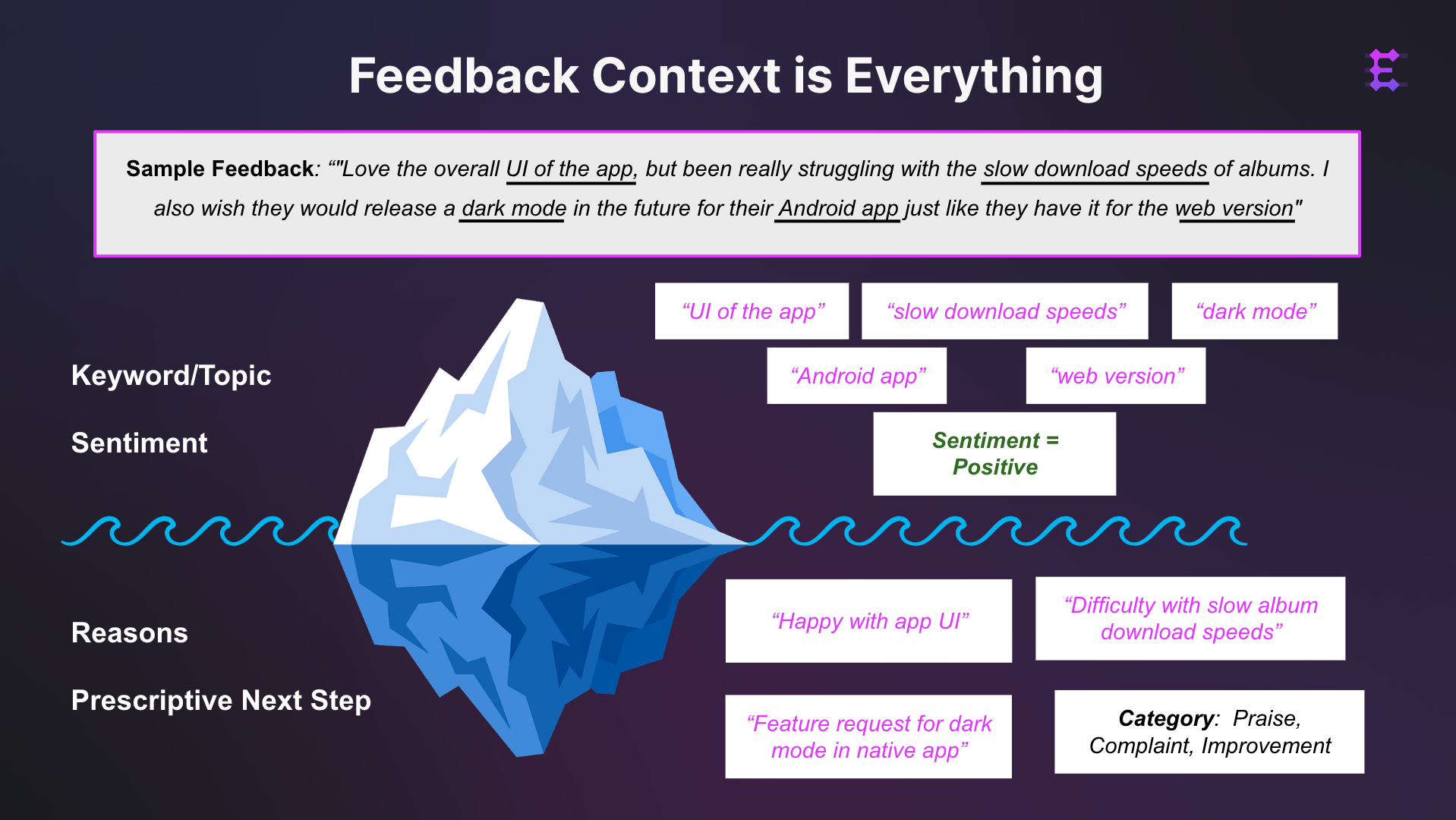
The best platforms go deeper. Hidden behind the keywords is the most important element; the context of a specific feedback record. Without this qualification, keywords are not particularly useful. Enterpret’s adaptive custom ML models analyze every single sentence of each feedback record, and summarize each one into a Reason.
Reasons are a game-changer. They are semantic summaries of each element of each record. In the above case, the taxonomy would categorize that feedback record into the following Reasons:
- “Happy with app UI”
- “Difficulty with slow album download speeds”
- “Feature request for dark mode in native app”
The model would also assign multiple categories to this record, likely Praise, complaint, and Feature Request, providing an unparalleled level of conclusive context.
This shifts the analysis paradigm; gleaning a depth of insights into the specific actionable next step that needs to be taken to address this issue. At scale, if you see 30% of feedback records noting difficulty with the login flow, you know there’s an issue, and you know what you need to fix.
Use AI for better granularity and quality for tagging feedback
"Ultimately, the biggest challenge in deploying machine learning isn't writing the code. It's in collecting and cleaning the training data. And more data beats a cleverer algorithm." - Andrew Ng, leading AI and Machine Learning professor at Stanford University
Machine Learning has brought feedback analytics from 0 to 1. While sophisticated algorithms are crucial, the value of machine learning ultimately hinges on the quality and quantity of the data used for training and, in the case of feedback, the level of granularity in tagging.
In short, the context of the feedback is just as important as the topics (and in some cases, more). If you aren’t examining that context, you likely will have major blind spots.
We can help - get in touch!