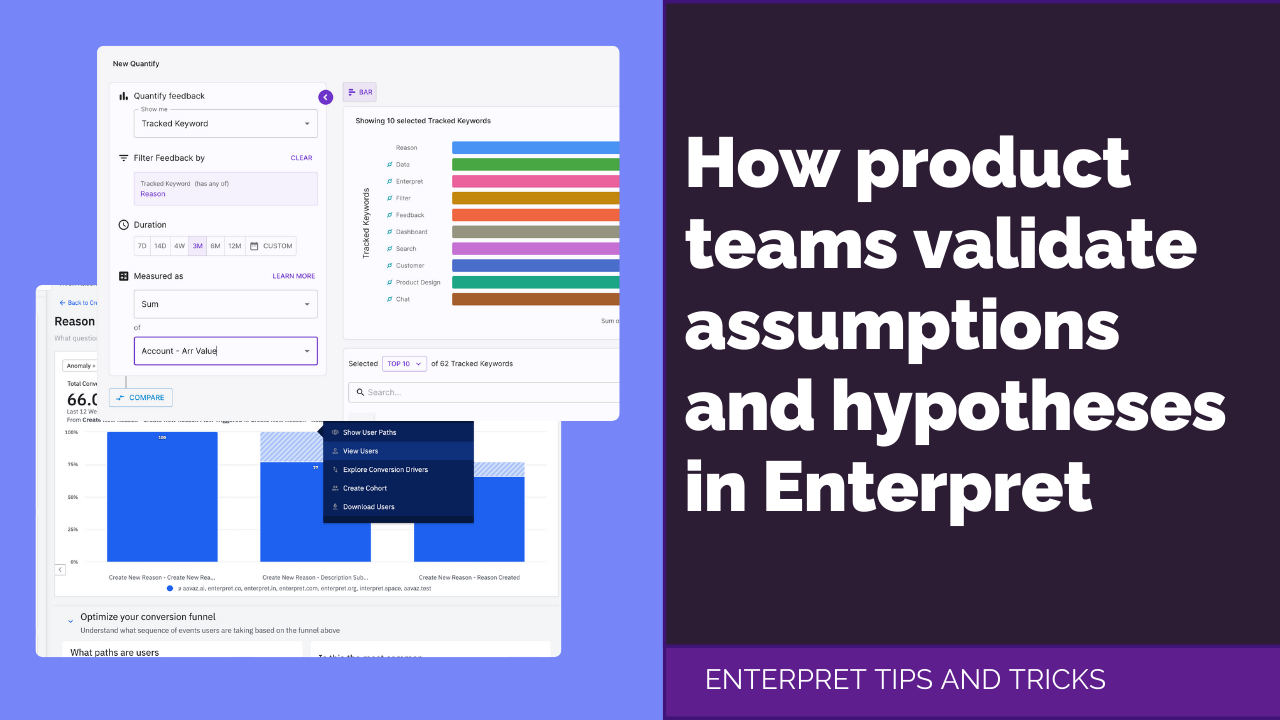
How product teams validate assumptions and hypotheses in Enterpret
.webp)
Hey there, I’m Vivek, and I’m a Product Manager at Enterpret!
At Enterpret we use Enterpret to build Enterpret. Our Product Team uses Enterpret in many ways, from helping us measure the success of launches to how we make product decisions.
One of the most important product use cases for Enterpret is the ability to validate our assumptions and gauge the success of our bets. Being able to join quantitative and qualitative feedback quickly helps us to automate our feedback loops so we can efficiently move our product forward.
In this post, I’ll share a short case study of how we iterated quickly after our recent Reason Creation Launch by validating our hypotheses in Enterpret.
Why we launched Creating Reasons in Enterpret
We launched Creating Reasons to solve a challenge our customers had when working with our Taxonomy. While having an LLM powered Taxonomy that automatically tags feedback saves time, it does not always address the challenges of:
- Customers talk about feedback in different ways than your internal teams
- Predicting the level of granularity your business wants to understand specific and important pieces of feedback
- Customers talk about the same problem in multiple different ways
In listening to our own customer feedback 😃 we realized that our users wanted the ability to define Reasons on top of the existing Taxonomy. Enter, Create Reasons. This functionality gives our users more agency and control to quantify and track areas of customer feedback in Enterpret.

Digging into the Create Reason Launch Results
There are three steps in Creating a Reason:
- Users describe the Reason they wish to create — we use this description to curate definitions and find matching feedback
- Users can then review curated definitions, add/remove definitions
- Users can confirm the definition and create Reason (success)
Here’s the funnel in Amplitude:
.png)
After launch we noticed a sharp drop-off in transitioning from step 1 to 2. With a 23% drop-off rate.
.png)
Digging into the Analytics and some Assumptions
We did not know exactly know why users are dropping off. After looking at the product feedbackl data our team had some working theories:
Blank-slate Problem — it’s difficult to start describing a Reason you want to create. This is intimidating to users, and they’re struggling to describe the Reason effectively.
- Problem to Solve: What would be more helpful instead?
- Assumption: We show examples of descriptions to fight the blank-slate problem. Are the examples not helpful? why? what can be better?
Just Checking Out — It’s a new feature, with a prominent product announcement. Users might very well be just checking the feature out, without an intention to actually create a new Reason.
Validating our Assumptions in Enterpret Using Feedback to Gain Clarity
Using the Enterpret <> Amplitude Integration we are able to sync over the cohort of 23% users.
.png)
In Enterpret we can zoom into the feedback from this cohort of users by creating a Search for:
- Feedback on Reasons
- There’s also the option where we can filter by user_email in {email addresses who dropped off}
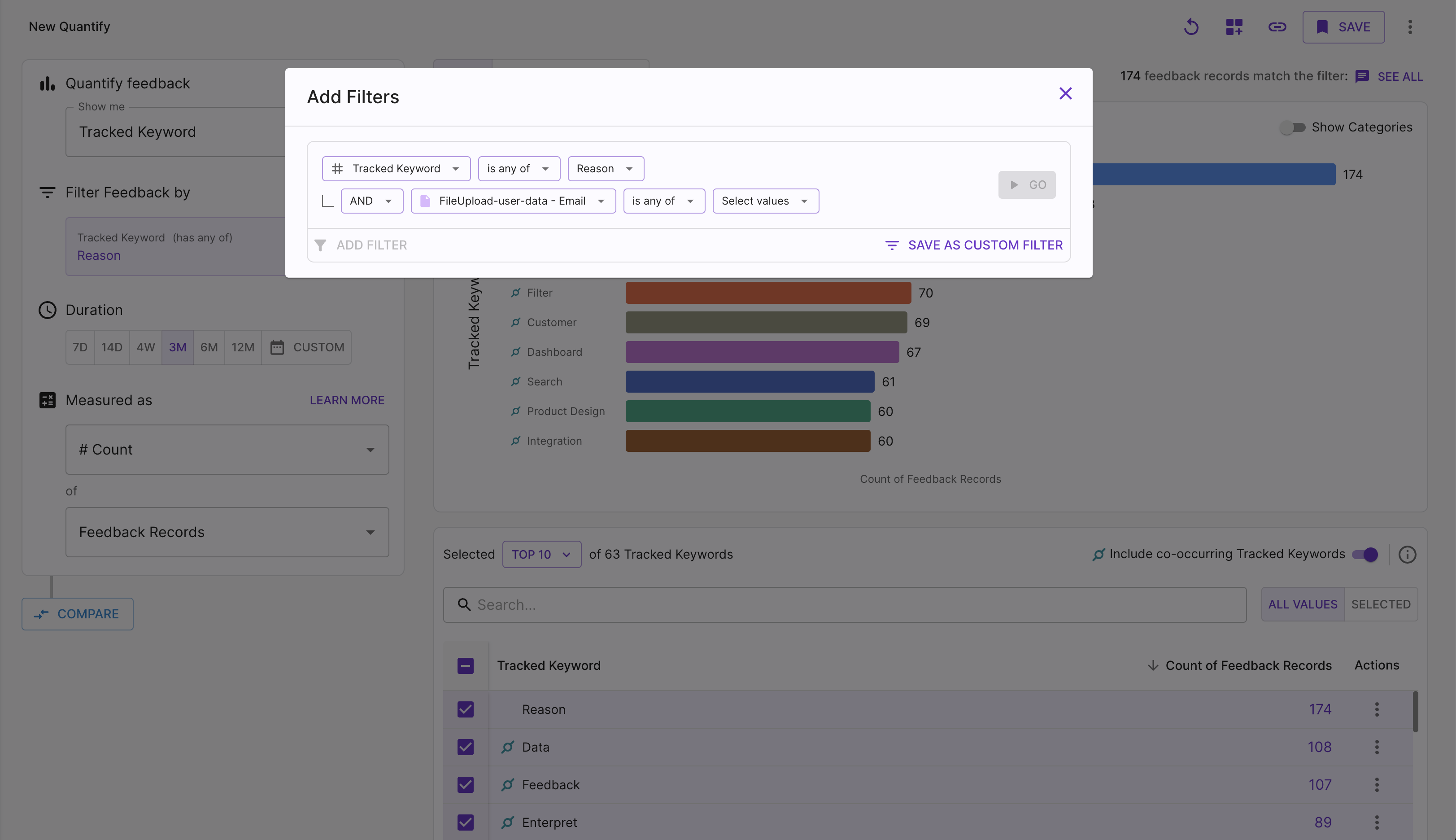
By zooming into the launch feedback our team is able to validate if our hypothesis of a blank-slate problem is true. We can also understand “why” it’s a problem, and start to answer questions of
- Why did providing Create Reason Examples not help?
- What could have been better?
Prioritizing Using Impact Analysis
Once we have our validated assumptions we take the next step of deciding whether the work gets prioritized by using Synced Users and Accounts.
Everything is a tradeoff. Synced Users and Accounts allows us to analyze the impact of addressing a particular issue on different customers and revenue.
%20(1).png)
I hope you found this case study on how product teams can validate assumptions and hypotheses in Enterpret helpful! If you have any questions feel free to reach out to me vivek@enterpret.com or find me on X @vi_kaushal!