
How to Analyze Customer Feedback
.webp)
Everyone realises the need to understand the voice of their customers but it’s incredibly challenging to do it successfully. Challenges include collecting all feedback together, discovering what tags to apply, tagging feedback correctly, finding and sharing insights with your teammates.
We, at Enterpret, have been working on building analytics on top of customer feedback to make sure you are able to learn from your customers and the feedback they share with you.
In this blog, I’m going to elucidate the needs and challenges of analyzing customer feedback and how can you do successfully. In the second half of this blog, I'd be sharing how Enterpret helps you analyze customer feedback with ease, and what’s different about Enterpret’s approach.
Why do we need to analyze customer feedback?
Adam Nash (VP, Product at Dropbox), in this wonderful presentation, talks about the importance of listening to customers. Customers have a relationship with your product and share feedback on how that relationship can be improved. If their voice is not heard, the relationship is jeopardized.
Customer feedback is important for product development and product quality. Proper analysis is imperative to get a better view of what has to change and improve in the product to provide value to your customers.
What makes analyzing customer feedback so difficult?
- Feedback is scattered: Today, customers give feedback in many different places and expect the product to respond and evolve to their feedback. Feedback could be shared in a support ticket, Slack channel, survey, as a review on G2 or an app store, or even User Interviews and Sales Calls. Moreover, feedback is multilingual for global products.
- Creating the Feedback Taxonomy - Identifying the tags: Properly analyzing feedback requires tagging each piece of feedback with topics, like ‘Subscription’, and the feedback reason, like ‘error when adding credit card’. This is extremely challenging for the following reasons:
→ Keywords could be mentioned in multiple ways by your customers. For example, subscription could be subscribed, subscriptions, subscribes, etc. You need to maintain a mapping of the topic name, and all the keyword variations of it.
→ Feedback reasons need to identify what the customer meant rather than what they said, i.e., semantically instead of syntactically. There could be infinite ways to say the same thing, but you want to group feedback with similar meanings together.
→ The number of important keywords and reasons is very high. Most products have hundreds of keywords and thousands of reasons contained in their customer feedback.
→ Taxonomy is an iterative process: You won’t know all the possible tags upfront. You will need to go through feedback, define what you want to tag, refine the tags, and repeat.
→ Your feedback taxonomy needs to evolve as your product, customers, geographies, etc. evolve.
- Applying the Feedback Taxonomy - Tagging each piece of feedback accurately: Even if you create a robust feedback taxonomy, you then need to ensure every piece of feedback gets the right tags. It is a rule of thumb in data annotation that accuracy is inversely proportional to the number of tags. Further, it won’t be just one person doing the tagging but many people — often customer support agents who trying to resolve tickets quickly. To maintain consistency, everyone who is tagging needs to have the same understanding of every scenario. Doing this for thousands of tags is nearly impossible. Tagging is time-consuming, resource intensive, and inherently inaccurate.
How to analyze customer feedback
There are two major aspects to analyzing customer feedback:
- Giving feedback a structure
- Understanding the context of feedback
Giving feedback a structure
Above, you can see feedback from a customer of Notion. The feedback contains the following keywords:
- Subscription
- Difficulty in adding a card
- Google Pay
- Error: wrong payment method
Consider all of the different keywords contained within your product feedback. You could have hundreds.
In addition, different customers will share feedback for the same reason, but use different wording to describe it (e.g. I can’t renew my subscription, getting a payment error on resubscription, etc.). There could be infinite ways to describe a reason for feedback. Furthermore, these reasons for feedback themselves could very well range into the thousands.
To give feedback a structure, you need to accurately identify different keywords and reasons. This structure is called the Feedback Taxonomy.
Once the feedback is structured and accurately tagged, then you can answer questions like the ones listed below to both find and quantify relevant feedback:
- How many times have users complained about wrong payment method through google pay?
- Has the volume of this feedback changed over time? What’s the trend?
- Have these users churned since giving that feedback?

Understanding the context of feedback
Surfacing themes of feedback is helpful, but what makes feedback truly valuable is understanding the context: who the customer is, what was their behaviour, and where and when they shared the feedback. Tying the feedback they shared with the context of who they are is critical to unlocking insights with real business value, as opposed to just a generic list of the top 5 feedback themes in your user base.
For example:
- To understand leading indicators of churn, you’ll want to isolate the feedback of churned customers and analyze feedback leading to churn.
- To investigate a particular user behavior, like free users who did not convert to a paid plan - what does the feedback shared by these users tell us?
.png)
How does Enterpret create your custom feedback taxonomy?
At its core, Enterpret uses custom large language models to build an automatic feedback taxonomy customized to your product.
Enterpret’s unified feedback repository has native integrations that connect with feedback sources where natural language interaction happens between you and your customers. The feedback repository ingests feedback in any language, translates non-English to English.
Model training is automated by fetching historical feedback. After fetching all historical feedback, Enterpret removes spam and junk, since support channels can get a lot of spam.
Once the data is clean, Enterpret projects the feedback into a semantic space by leveraging the large language models I mentioned above. In the semantic space, all similar meaning text is clustered together. We then group these clusters, and give each a name — these are your feedback reasons. Let’s look at an example:

Let’s look at a real examples:
- “As a paid premium user since 2011, it’s time to quit @evernote and switch to @NotionHQ”
- “Moved from @evernote to @NotionHQ today!”
- “I stopped using Trello and Evernote”
All three of these tweets are essentially talking about the same thing - “Switching From Evernote to Notion”.
After cleaning, all three pieces of text would be extremely close in the semantic space and would get clustered together - and can be named the same repeatable summary. Switching From Evernote to Notion would get recorded as a reason for the above feedback and any other similar feedback.
Similarly, tracked keywords like Evernote, Trello, Todoist, Web App, etc will get identified and tagged on the feedback.
Enterpret scans through all your feedback, historic and ongoing, and identifies the major reasons and entities within your feedback. This identification goes through multiple checks, including a human auditor, to ensure uniqueness (”switching from Evernote” and “moving over to Notion from Evernote” mean the same thing) and relevance (making sure the feedback is about your product).


We do a taxonomy refresh at regular intervals so that new reasons and keywords get created as your product evolves.
As a result, Enterpret is automatically able to identify all the thousands of reasons for feedback for your product and hundreds of keywords relevant to your product - through no effort on your end.
How does Enterpret apply the taxonomy to your feedback?
After the taxonomy is created, Enterpret then tags each piece of feedback ingested from the Unified Feedback Repository against the entire Taxonomy.
.png)
We train a custom model on your data to accurately tag the entire taxonomy on each feedback record to get optimum performance. These custom models are essential for analyzing customer feedback. While off-the-shelf models like GPT-3 can perform well on Internet data as that is what they are mostly trained on, they will perform poorly on a custom data set like your product’s customer feedback.
In addition, we have a team of human auditors who constantly check the performance of your model’s predictions to ensure nothing has gone astray.
Models are probabilistic by nature and will have a few incorrect predictions. We guarantee state-of-the-art performance, but incorrect predictions are bound to happen. Whenever you notice a mistake, you can report that feedback within Enterpret, and the model will update to ensure the same kind of mistake isn’t repeated.

How do you get insights from customer feedback?
Ingesting all feedback, creating a Taxonomy, and then applying the Taxonomy - creates your data of feedback records. Enterpret then provides you with an interface to perform analytical queries and search for feedback.

Some sample questions you could answer using Enterpret include:
- Find feedback for your feature.
- What are the top reasons for feedback for my feature?
- Which reason for feedback for my product changed the most in the last month?
- Is there a particular kind of feedback present, and can I go see it in the context of where it was shared?
- What was the feedback shared by a customer segment and how is it different from another segment?
- We just launched a new feature; what feedback am I getting for it on Twitter?
- What reasons for feedback correspond more with lower NPS or CSAT or AppStore ratings?
…and many more.
How do you take action on top of it?
Here are a few ways you can leverage the insights you identify in Enterpret in your day-to-day work:
- Search for feedback, create a report out of it, and share it with your team.
- Create feedback alerts, which notify you as soon as any relevant feedback comes in.
- Set notifications for what’s trending up and what’s trending down in customer feedback for your feature.
- Monitor the change in sentiment of a feature after launching an update.
- As a salesperson or customer success representative, brush up on feedback before a call with your account.
- Find the users who shared feedback on the feature you’re working on, and reach out to them for user interviews.
- Find the users who asked for a particular feature or improvement, and let them know once the feature is live.
What is different about Enterpret’s approach?
Enterpret is differentiated from similar tools or generic models as it offers the following capabilities:
- Minimal onboarding effort: Enterpret’s unsupervised feedback taxonomy requires no training data or manual tagging effort. All you have to provide is access to your data. We are SOC-II compliant - so be rest assured, your data would be safe with us.
- Model fine-tuned for you: Every product is unique, with different features, capabilities, and taxonomy. With Enterpret we leverage our unsupervised clustering algorithm to identify the right keywords and reasons for you. Other tools either have fixed categories defined for every product or ask you to come up with the tags.
- Monthly model refreshes: As your product evolves, so does the feedback being shared by your customers. Enterpret reruns automatic detection on data that was not tagged to find new reasons for feedback. New reasons are then backfilled into historical data to ensure no customer pain goes unnoticed.
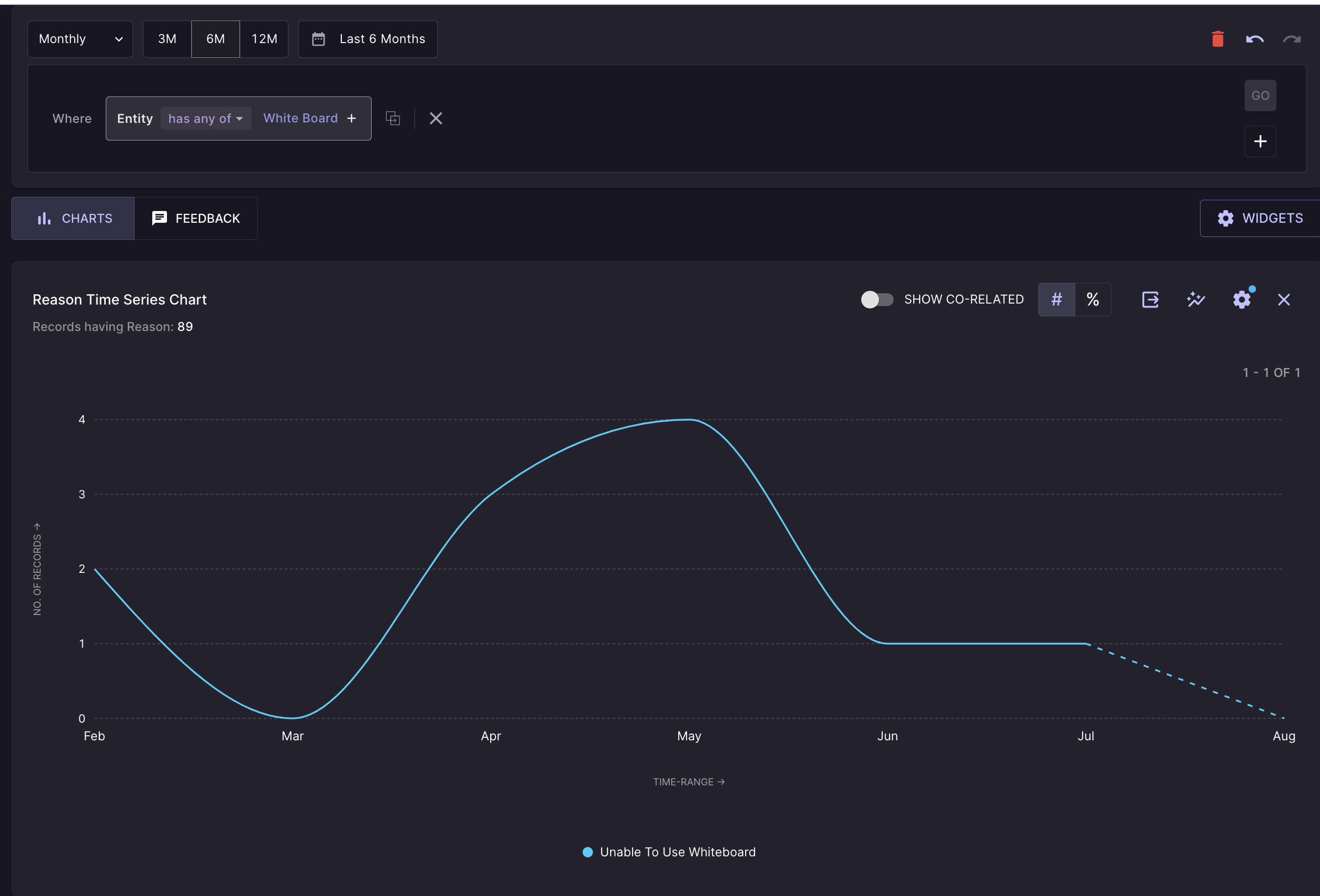
- Interpretability: For every prediction, see why and where in the feedback the prediction was made.
.png)
- Human in the loop: Enterpret has internal auditors who ensure that every new reason/tracked keyword detected goes through a quality check.
- Context: Along with what the feedback is, it matters who the feedback is from. Enterpret builds a dynamic user model from the feedback it ingests. So, you can see all the feedback whether in ZenDesk or Delighted submitted by a user or an account. You can also filter the feedback by a cohort or segment, and contrast it against another cohort.
Hopefully, this post shed some light on how we’re approaching the tricky problem of analyzing customer feedback.
We’re working with some great product and product ops teams like Notion, Figma, and Apollo.io to help them identify actionable insights to build better products for their customers.
If you’d like to learn more about how your team can use Enterpret, please reach out!